This article has been reproduced from the content published by a DiVoMiner user, which effectively explains the process of content analysis in DiVoMiner platform.
I believe that many undergraduate and graduate students have encountered the challenges of writing academic papers. The difficulties with academic paper go beyond simply reviewing literature, selecting topics, and establishing hypotheses. It also encompasses the complex task of data management and analysis.
Can the successful completion of your research be hindered by limited knowledge of Python, SPSS, or unfamiliarity with the steps of analysis? It should not be! In fact, there are various user-friendly platforms and software specifically designed for academic researchers, waiting to be discovered and utilized, ensuring a smooth research journey.
Content analysis has always been one of the most popular research methods across various fields, such as communication studies. If you want to analyze how media reports construct certain groups, cities, topics, etc., conduct sentiment analysis on trending topics discussed on social networks like Twitter, Facebook, TikTok, X etc., streamline the time spent on editing, storing, and organizing during the research process, or easily conduct cross-analysis of system variables and custom variables after coding, the following article will assist you along the research journey.
This article will take you on an immersive journey to master DiVoMiner, an AI-powered content analysis platform, which solves the typical challenges in content analysis.
This platform is tailor-made for content analysis, utilizing a combination of machine learning coding and human-machine interaction workflow. It enables the completion of the entire content analysis process online through cloud-based system. Database construction, sampling, coding, statistics, and result visualization can all be achieved with just a few clicks, saving time and effort!
Get a glimpse of what this platform can do to help you out
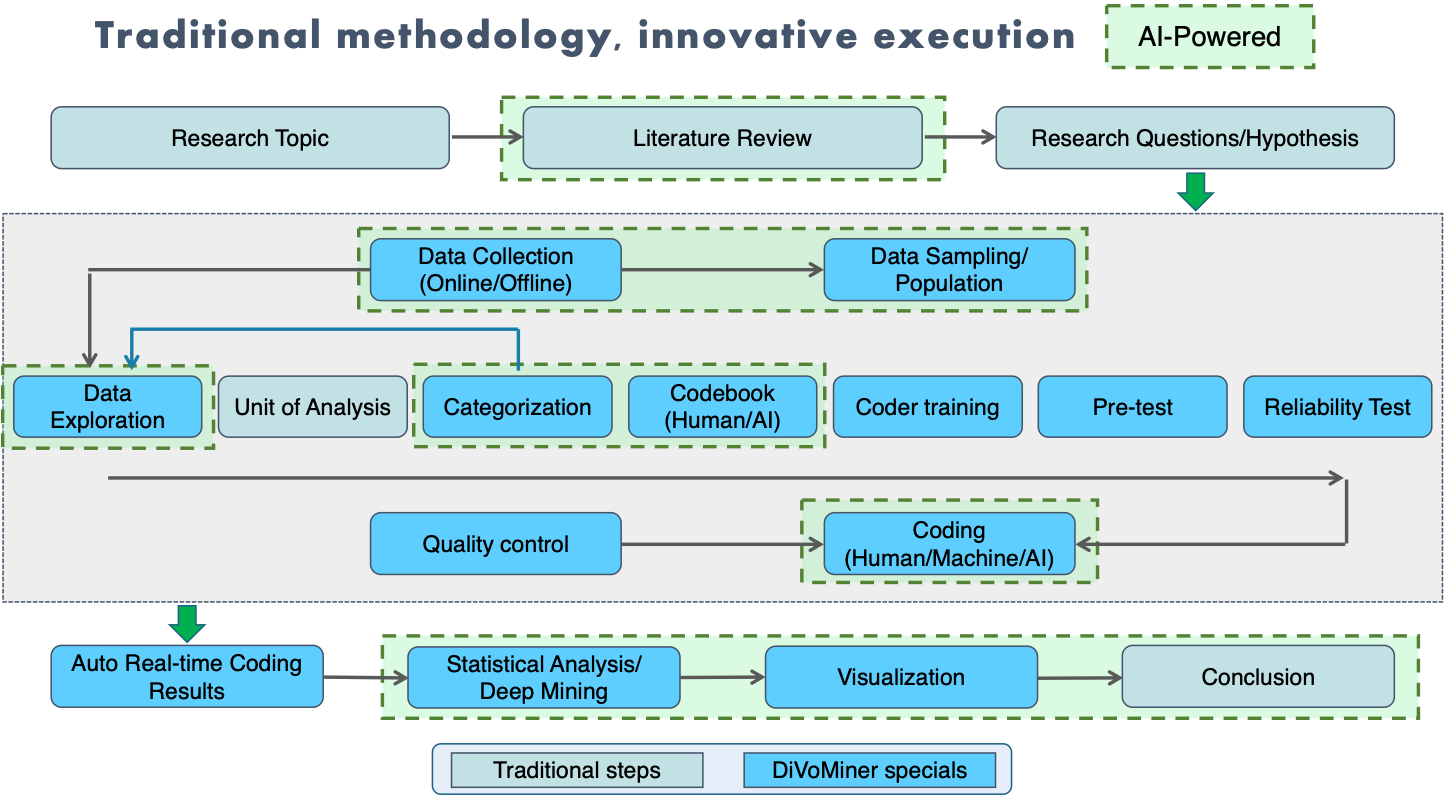
Now, let’s walk you through the operations of this platform in the following sequence:
Login > Upload Data > Sampling > Codebook Generation > Reliability Test > Coding > Statistical Analysis.
Login
No need to download any software, you can simply search for DiVoMiner in your browser, register for a free account. With the free account, you can utilize majority of the platform’s features. With DiVoMiner, research processes have never been easier.
Click here to have your DiVoMiner account for free!
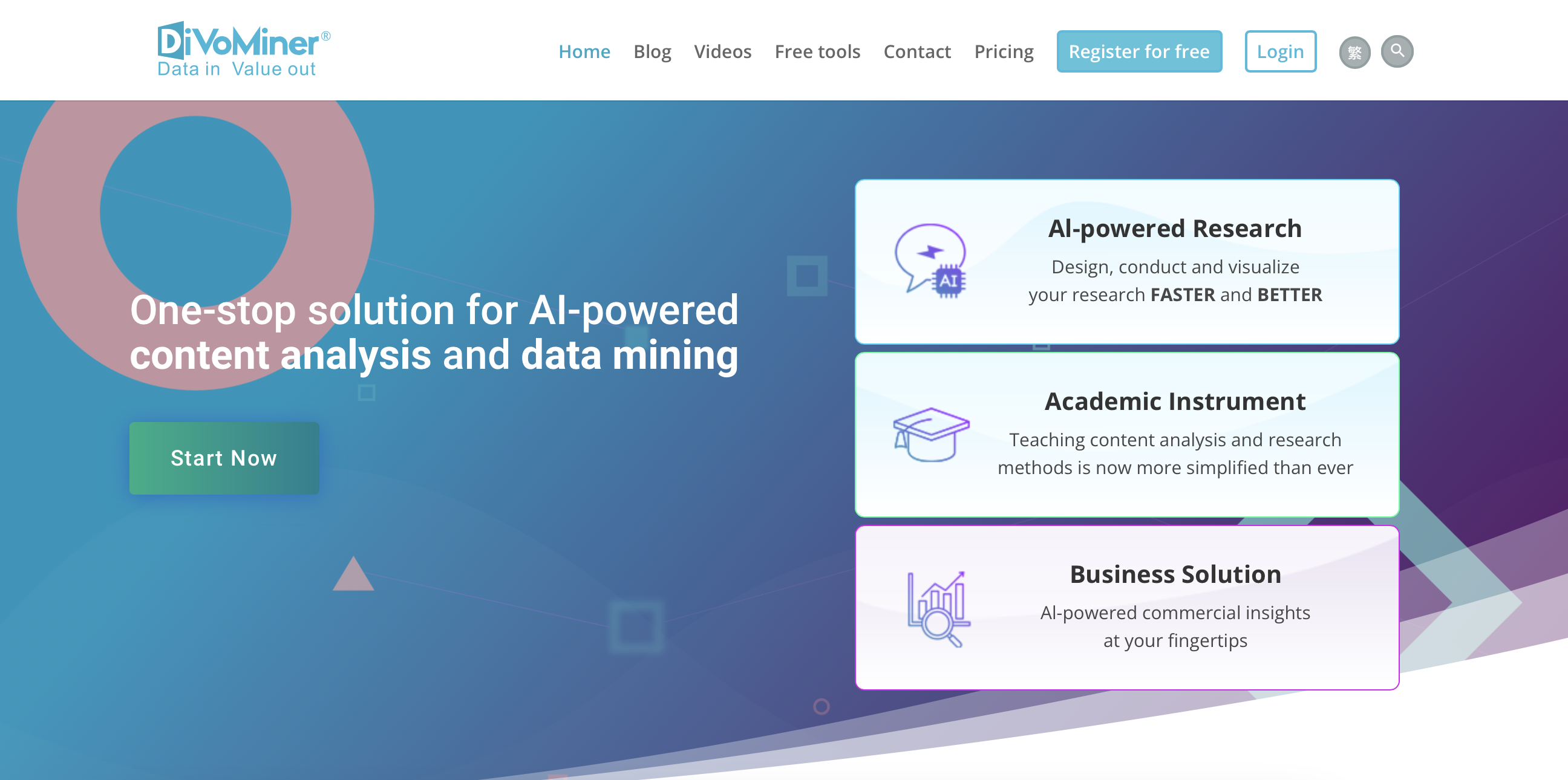
Upload Data
Prepare the research data sample objects that need to be coded in advance and organize it into a document. Create a free project in the homepage of the platform. Once you enter the project, you will find that this platform is really user-friendly. It perfectly captures the rhythm of content analysis.
Afterwards, you can add a database in the [Data Management] section and upload data in various formats. This includes Excel/CSV/PDF/Word/Text, as well as image, audio and video format.
Please note that the free version has limits on data and file capacity, which are 1000 entries and 50MB. If you need to analyze more data, you can also purchase additional resources on the platform.
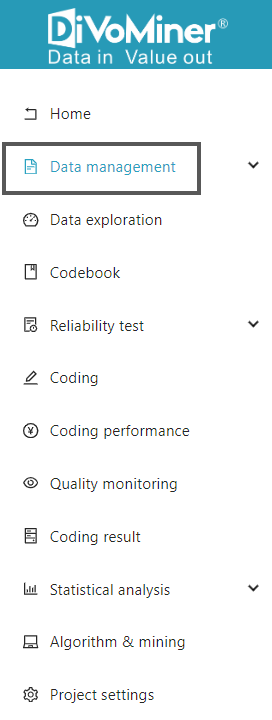
Sampling
If you have a sampling requirement for the data already uploaded, you can find the sampling option below the database. You can then choose to sample by proportion or by quantity, and the system will automatically generate a new sample library based on the sampling results.
In addition, this platform can also help you with coding using the power of technology. The AI-powered system can automatically identify keywords you set and code them. However, you can choose not to use this feature and only perform manual coding if you prefer.
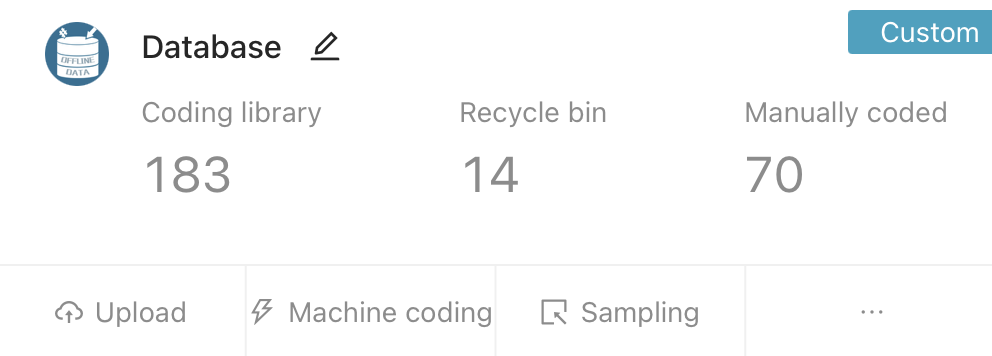
Codebook Generation
Creating a codebook is an essential step in the research process. Before this step, it is necessary to finalize the categories for the codebook. In the [Codebook] section, kindly input your questions and corresponding options. You may also add keywords to the options. These keywords will be highlighted during the coding process, serving as prompts for machine coding (this will be reflected in the coding section). This procedural approach is similar to the process of designing a survey.
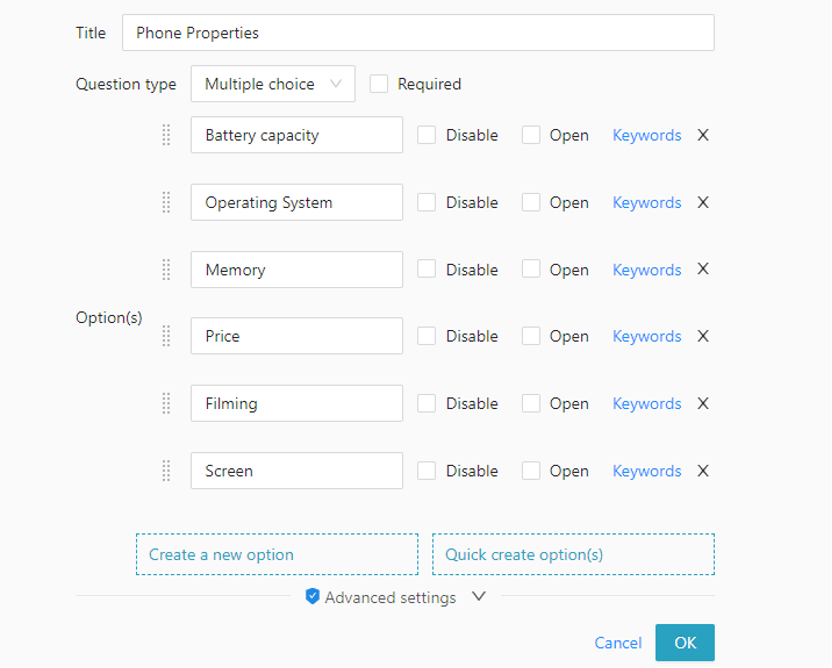
The DiVoMiner platform now offers AI-aided category generation along with the options based on research objectives which can be later imported to the Codebook. This amazing feature can be accessed from the ‘AI-generated category’ tab. To experience the full potential of this powerful tool, follow this in the DiVoMiner platform:

With the AI-generated categories, researchers can quickly identify the most relevant themes and features within their data, allowing them to focus on the areas that are most important for their research objectives.
Reliability Test
The next step involves conducting an inter-coder reliability test. It is advisable to randomly select a subset of samples from the [Coding Library] and import them into the [Test Library]. Subsequently, the coders should carry out the coding process. Following this, the inter-coder reliability can be calculated to determine if it meets the predefined standard before proceeding with the actual coding phase. There are four reliability indices available for selection, and you also have the option to calculate the reliability of specific coders or certain categories. Moreover, If machine coding is implemented, reliability test between coder and machine can be performed in the platform as well.
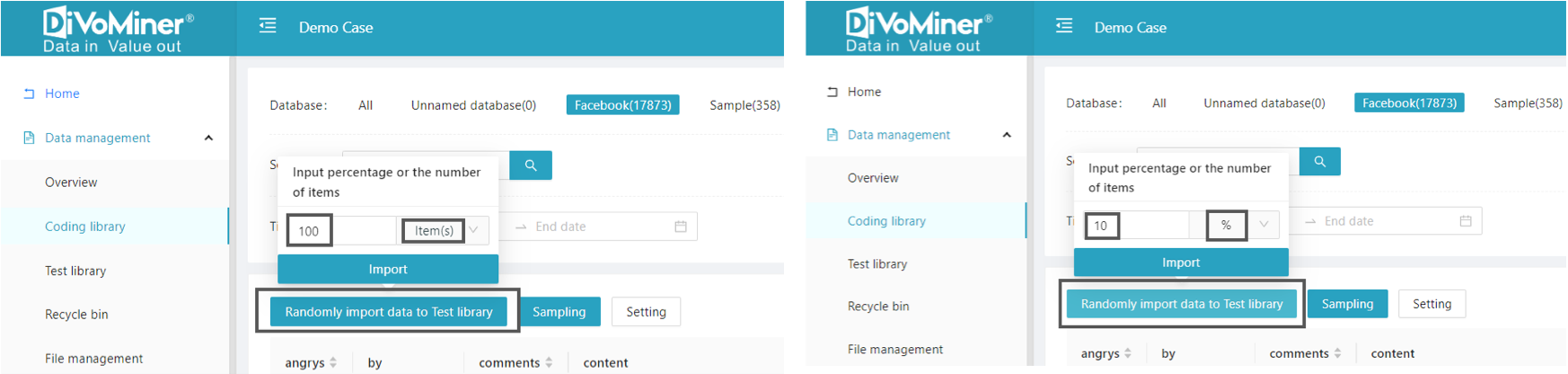
To extend an invitation to coders for project participation, kindly navigate to the homepage and locate the three dots positioned on the right side of [My Project]. Subsequently, you may select the “Invite” option to extend invitations to others and invite them to join the project.

Coding
The process of coding can also be considered as the coders “filling out a survey” for each data entry. The data content is presented on the left side, while the coding categories are on the right side for easy comparison. You can review the data you have previously coded and leave comments on specific data entries for future reference. Notably, this system offers the remarkable feature of assessing coding performance and monitor the quality of coding. The project administrator can easily track the progress of each coder’s work.
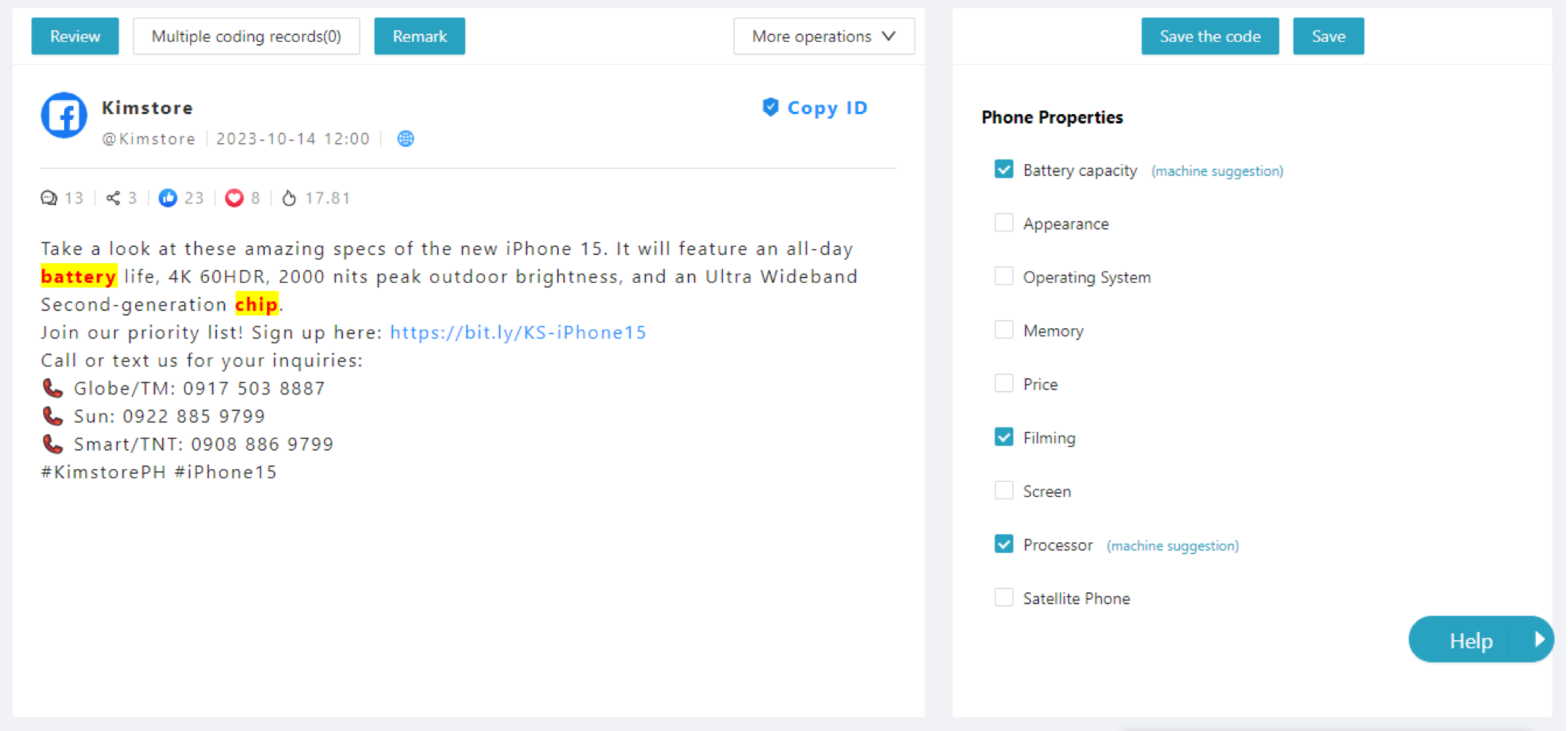
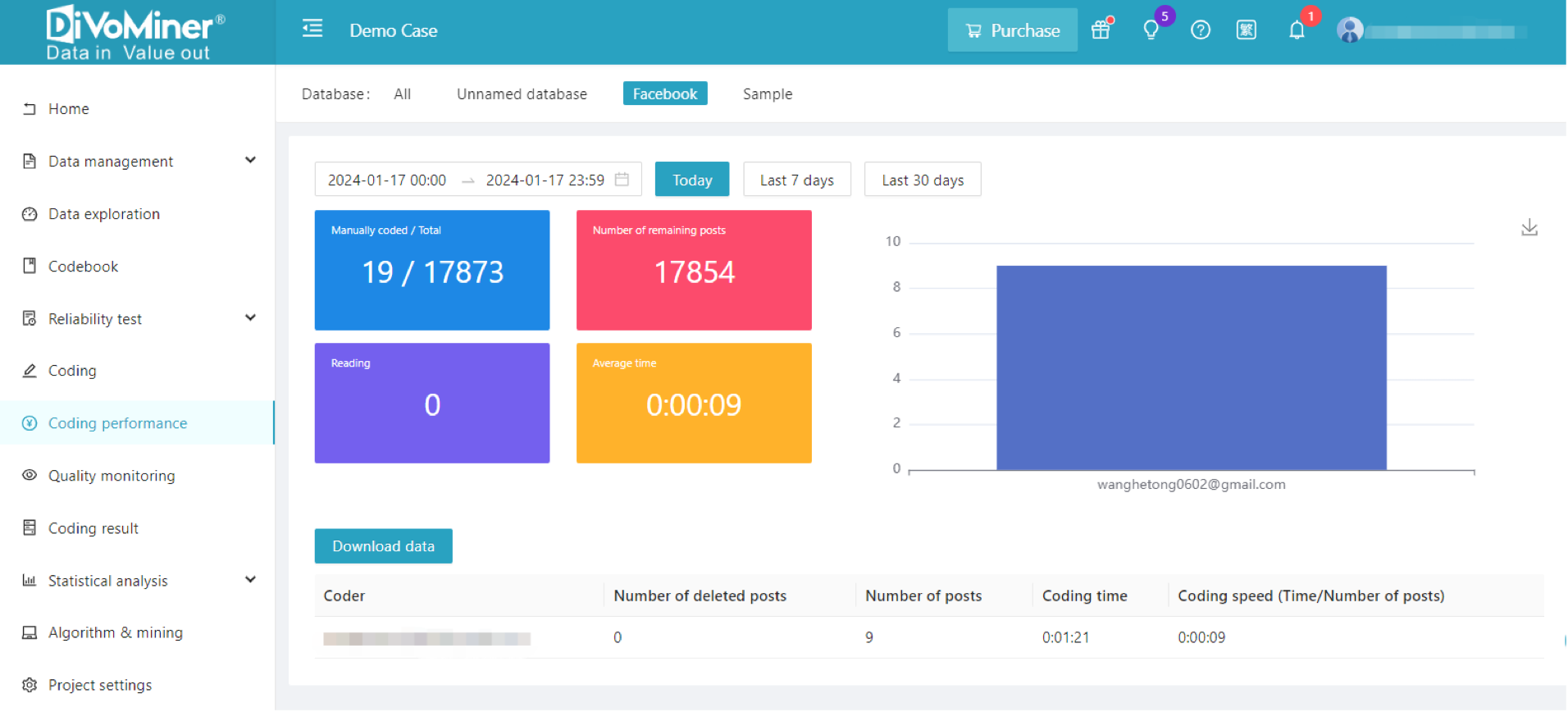
Statistical Analysis
After the coding is completed, the next step is to view the coding results and visualize them, which is crucial for analysis and report/paper writing. The platform allows you to easily create different types of charts by dragging and dropping variables. You can also select the dimensions and values for analysis. Statistical modules like descriptive statistics, correlation analysis, and chi-square are available for direct use. You can export the charts and coding results with just a few clicks. This article provides only a partial demonstration. For more features and algorithms, please visit the official website of DiVoMiner at https://me.divominer.com/.
(Following images are from the Demo Project in DiVoMiner)
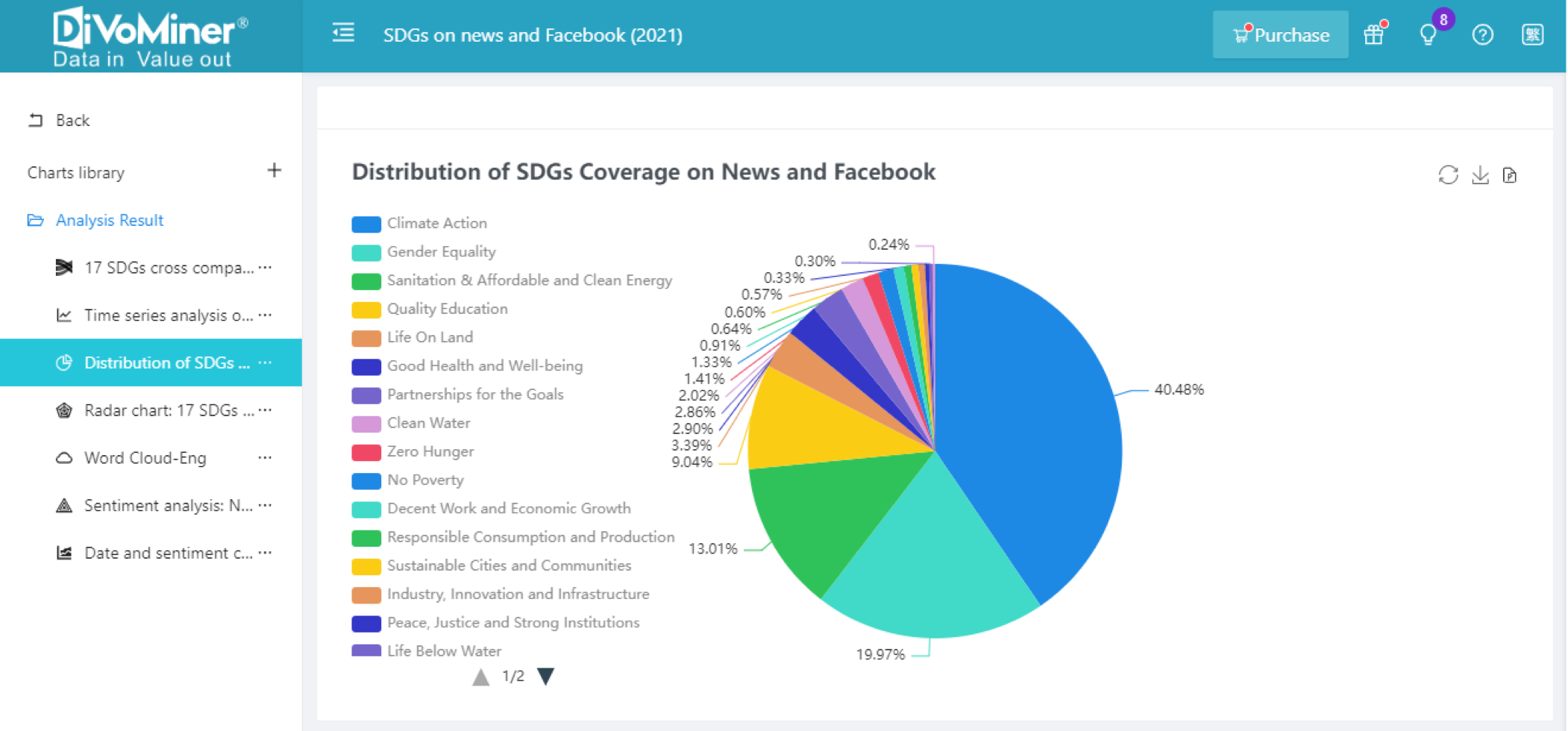
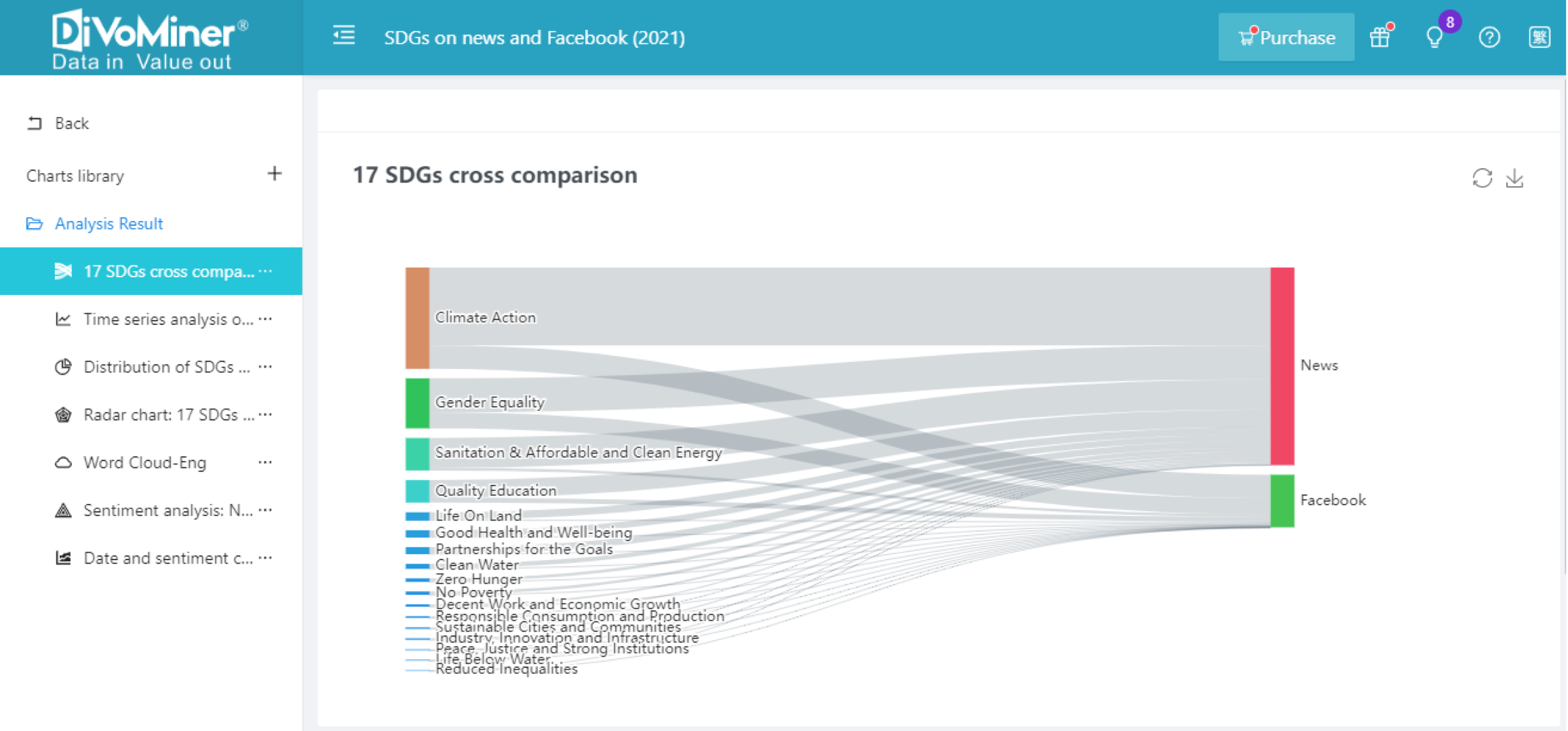
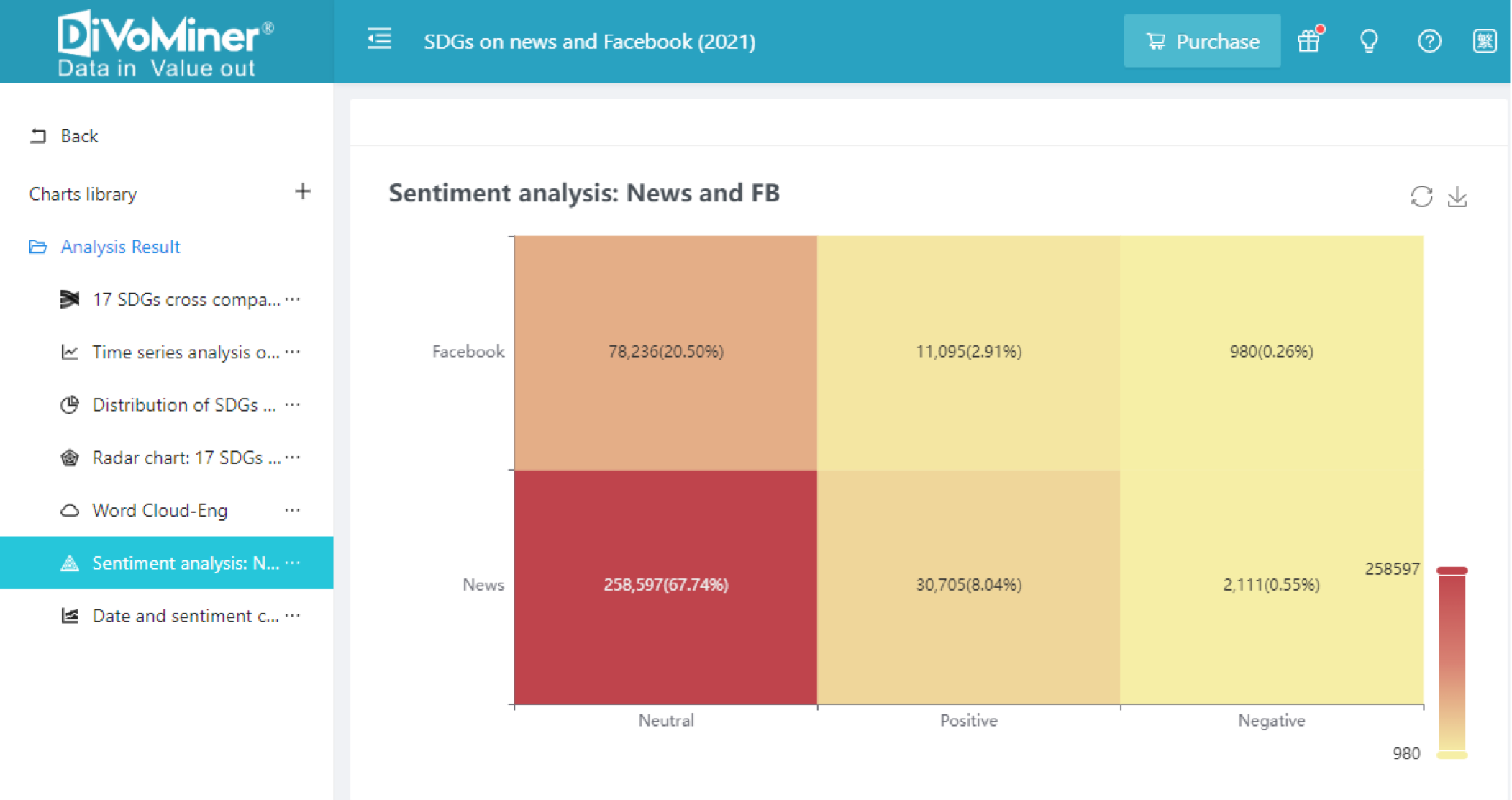
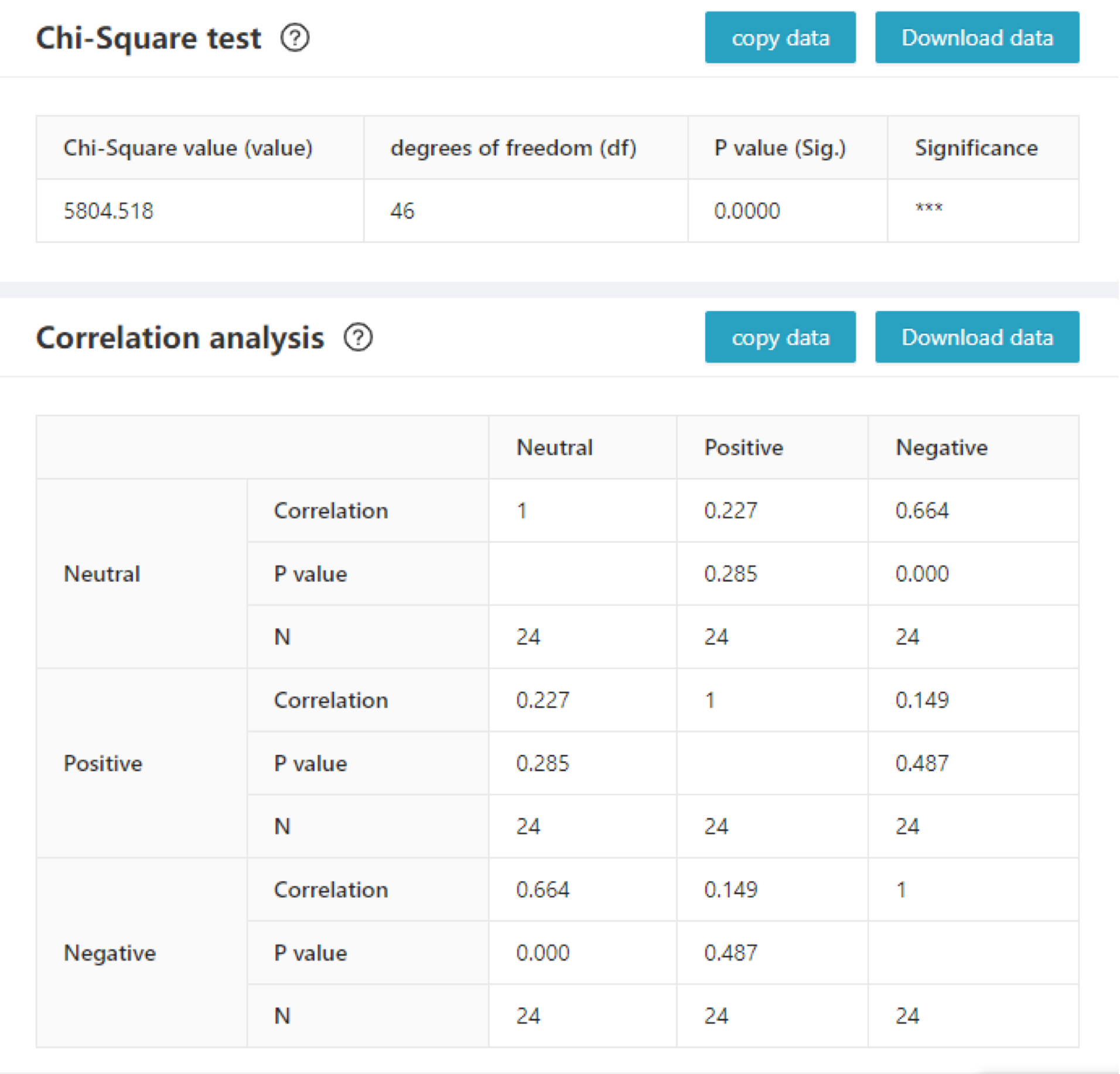
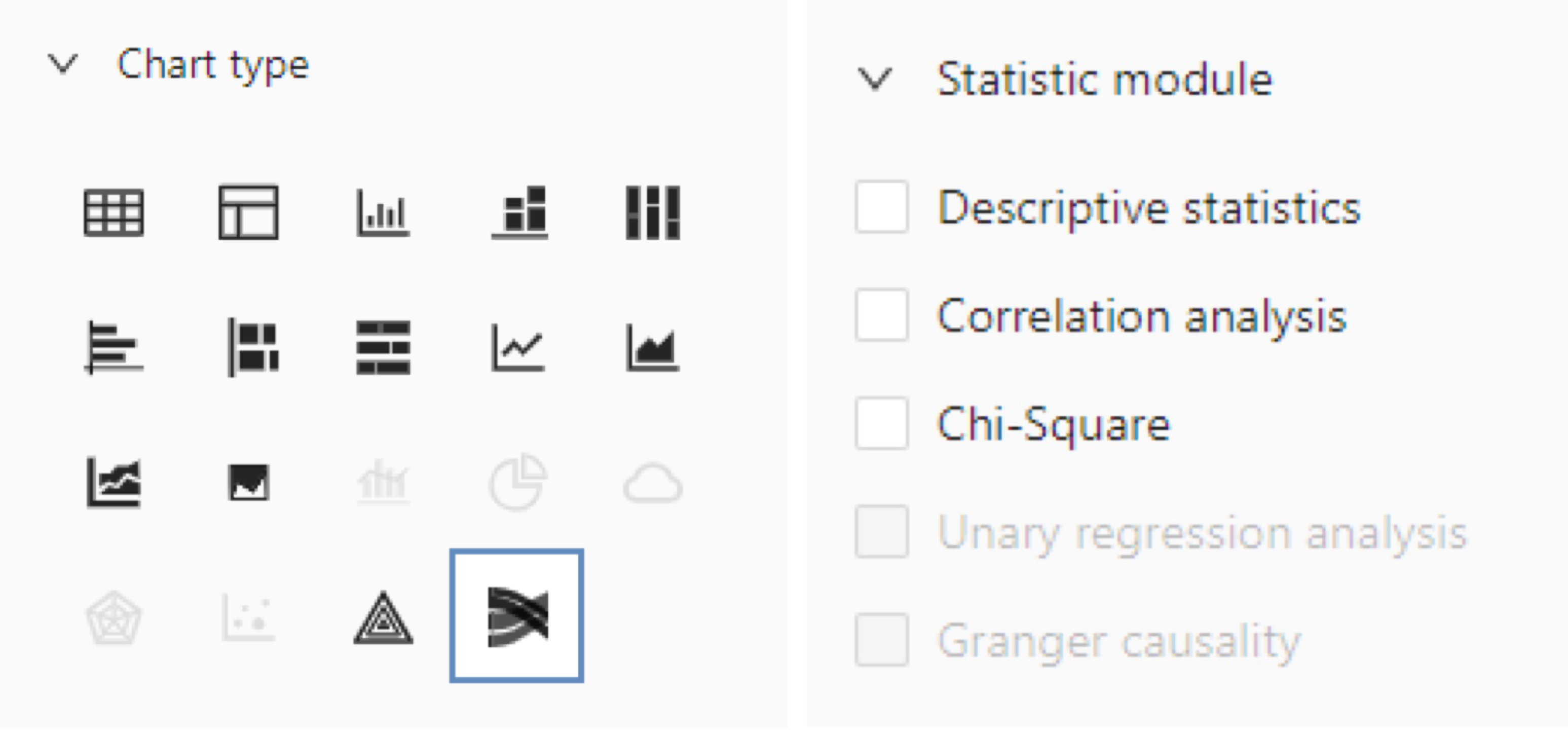
Still confused? Check out DiVoMiner’s video guides, and your research ideas will be clearer! The video section has complete guide to the platform demonstrating how efficiently you can conduct content analysis in DiVoMiner.
Video guides: https://www.divominer.com/en/videos/
After learning about all these information, does it make you eager to try it out? Just visit their official website at https://www.divominer.com/en/ and create your free account to get started.